General Motors validates digital twin of GMAW simulations to reduce distortions
by Fernando Okigami, Technical Enablement Manager, Hexagon’s Manufacturing Intelligence division
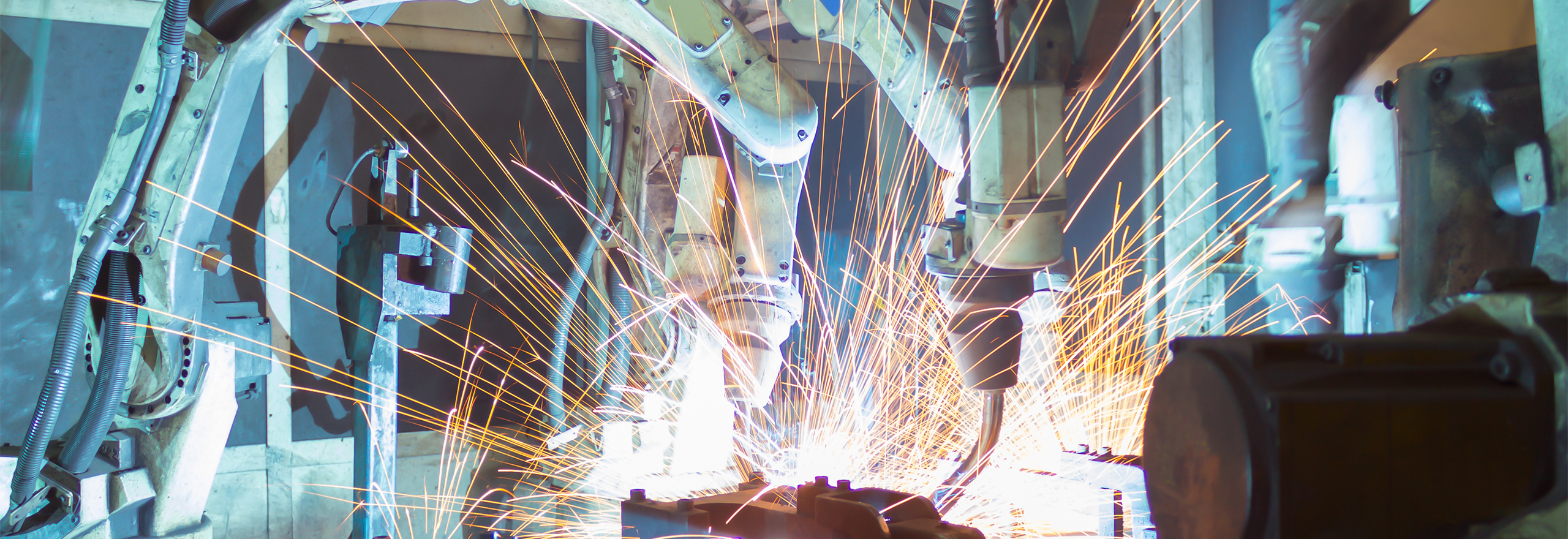
Engineering Reality 2023 volume 2 edition
Empowering Makers for an autonomous sustainable future
General Motors is a global company focused on advancing an all-electric future that is inclusive and accessible to all. It’s known for its long history as an automaker and selling vehicles under the Chevrolet, Buick, GMC, and Cadillac brands.
When building a vehicle, there’s little margin for error. Welding-induced distortion is a major concern in the assembly of automotive components. Distortions and other dimensional/structural integrity issues discovered in the manufacturing process slow down production and increase the rework needed — ultimately leading to increased costs and waste.
Gas Metal Arc Welding (GMAW) is a welding process in which an electric arc forms between a consumable wire and the workpiece. The arc heats the workpiece locally to the melting point leading to a permanent joint. The process is relatively fast, very controllable and produces sound welds. As with any joining process, the thermo-mechanical behaviour of the materials involved will determine the weld quality. Therefore, it should be carefully considered to avoid excessive distortion and detrimental residual stresses that impact dimensional and structural integrity.
Thermal distortions during welding also highly depend on fixturing conditions of the workpiece, welding sequence, and welding parameters such as heat input and welding speed. While there are methods to reduce distortion, such as not over-welding, it is challenging to account for all the variables that could affect the GMAW process. It often comes down to the operator’s skill, multiple physical experiments, and a time-consuming trial-and-error approach.
Figure 1. Straight-clamshell assembly – CAD model.
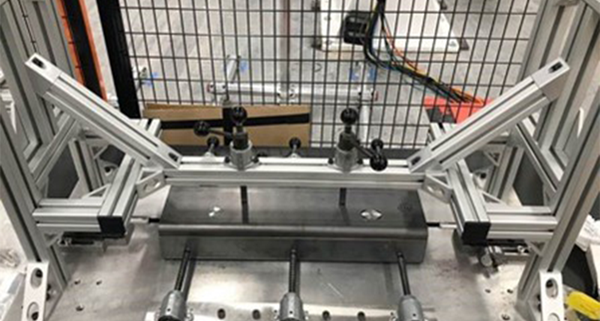
Figure 2. Straight-clamshell assembly – actual fixture.

Figure 3. Straight-clamshell assembly consists of two U-section channels (A1011 HSLA steel Grade 50) joined by two welding robots.
Test set-up to simplify welding simulation
Finite element-based welding simulation plays a vital role in predicting distortion so that the welding process can be better defined during the design phase to alleviate the issues experienced in production. However, welding simulation is a complex multiphysics problem subjected to many variables. It is highly nonlinear and involves the weld parts’ phase transformation and plastic strain. To meet this challenge, the research team from General Motors set out to validate its use of simulations applied to the GMAW process to address distortion issues.
General Motors researchers and several welding specialists from Hexagon worked together to create and validate a digital twin with a physical assembly for the GMAW process. The welding simulation of a clamshell comprised of two U-channels was studied under various process conditions such as clamping-unclamping process design, pre-bending, and tack welding, the number of weld segments, welding sequence, and direction. This evaluation study investigated the effects of heat sink by fixture, preheating of components, local joints, and contact behaviour of the many involved parts on welding distortion.
A total of eight validation tests were performed under different welding conditions, two representative cases captured the essence of the testing. For Case 1, the U-channels were fully clamped at 8 points without tack welds. For Case 2, the top 2 clamps were removed after tack welding in the four corners. The welding parameters applied were 22.0 volts, 185.0 amps, 10.0 mm/s speed, and 1.2 mm filler wire diameter.
Validating the accuracy of these virtual tests is of primary importance, thereby reducing the need for physical testing. A Hexagon 3D imaging laser scanner was used to compare shapes of physical parts with the results from the numerical simulation.
Load cells were attached to the clamps to measure weld-induced reaction forces, and thermocouples were attached to assess temperature distribution in the welded assembly. This model was then replicated virtually using Hexagon’s Simufact Welding, a software tool that gives the user a good level of flexibility while keeping the modelling process simple. This tool enables the user to run multiple virtual tests with only minor changes between each.
Validating the simulation results involved looking at the performance of the virtual models compared to physical experiments under the same conditions. General Motors validated the results across four sets of parameters to ensure accuracy.
Temperature
Accurately predicting temperature gradients in the assembly during welding is the most critical step for the numerical simulation of welding. GM identified 12 reference points on the weldment assembly to compare numerical simulation with physical data. The team found that the simulated temperature histories predicted agreed very well with the measured temperatures.
Displacement distribution
Steep temperature gradients witnessed during welding introduce part distortion. To validate the numerical simulation results with actual welding test data, the team used 3D scans to measure the displacement. They started by using the Hexagon 3D imaging laser scanner on the upper and lower channels of the assembly before welding. After welding, the team scanned the weldment assembly again using the same scanner.
That scan gave the team enough information to compare against the simulations, finding they agreed. In Case 1, the team observed relatively large deformations (~8 mm) near the upper channel corners where the welding terminates. Very small or almost no deformation was observed in the lower channel, especially near the clamping areas.
For Case 2, the deformations in the upper channel were larger near the edges compared to those in Case 1, which can be expected as the upper and lower channels are tack welded before welding, and the upper channel is not clamped during welding. The maximum distortion location and magnitude (~1.5 mm) predicted by simulation were found to match very well with actual welding test measurements for Cases 1 and 2.
Figure 4. Locations of 12 thermocouples.
Figure 5. Temperature distribution simulation for two use cases.
Figure 7. Displacement field for Case 2.
Load cell measurements
The team measured the clamping forces caused by distortion using load cells during welding. These findings helped conclude that the predicted reaction forces from the numerical simulation agreed with the actual measurements.
Figure 8. Maximum distortion for Case 1 .
Weld penetration
One of the most important steps when performing a welding simulation is to ensure the weld profile is similar to the physical one. The result of the thermal input will dictate the structure’s response to distortion and stress.
In a physical study, the team validated the simulation’s accuracy, where two robots performed a weld on parts in a rigid fixture. The simulation model’s weld penetration was accurately captured despite not reflecting all the macrograph variations.
Figure 10. Optical microscopic images of actual welds for (a) Case 1 and (b) Case 2.
Figure 11. Weld penetration simulation for Case 1.
Distortion results
These tests provided a better understanding of Simufact Welding’s modelling and simulation capability for predicting welding-induced distortion. This also gives users greater confidence in the ability of the software to evaluate and mitigate weld-induced distortion during the design phase – this leads to fewer physical tests, less rework, and reduced waste.
Simulations of transient temperature gradients due to heat input under various welding sequences matched very well with thermocouple measurements. Accurate prediction of the thermal behaviour of an assembly is a prerequisite to the simulation of welding distortion.
The simulated distortions of the assembly under various welding conditions were in excellent agreement with the scan data.
Simulation results were compared with the clamping forces measured using load cells during welding tests. The team found an excellent agreement between simulation and test data. Welding distortion is also greatly affected by tack welds. Without tack welds, the maximum distortion was more than 8.0 mm at one location, whereas the distortion was reduced to about 2.0 mm with properly placed tack welds.
Takeaway points
Overall, this work provided a better understanding of commercial software’s modelling and simulation capability for predicting welding-induced distortion. Simufact Welding can be used as a guideline for accurate simulation of welding processes. The results helped GM gain greater confidence in the ability of the software to evaluate and mitigate weld-induced distortion during the design phase far in advance of production.
As more businesses look to simulations and virtual testing, they must take the necessary steps to ensure accuracy. Using 3D imaging and numerical simulations ensures each test will be as accurate as possible. General Motors found Simufact Welding incredibly accurate when replicating real-world conditions.
GM engineers can use computer simulations instead of spending weeks on the shop floor to get the fixture and weld sequence so they understand what they’re getting straight off the computer. The result is a dramatic reduction of prototypes, leading directly to cost and time savings. These learnings from GM and Hexagon are important to the manufacturing industry because they can guide future simulations.
Figure 12. Comparison of data from the experiment and simulation.