Bosch Group slashes costs, reduces calibration time with ODYSSEE CAE
By Camilo Cruz, Research Scientist in Plastics Engineering, Robert Bosch GmbH, and Sandra Saad, Development Engineer, Robert Bosch GmbH
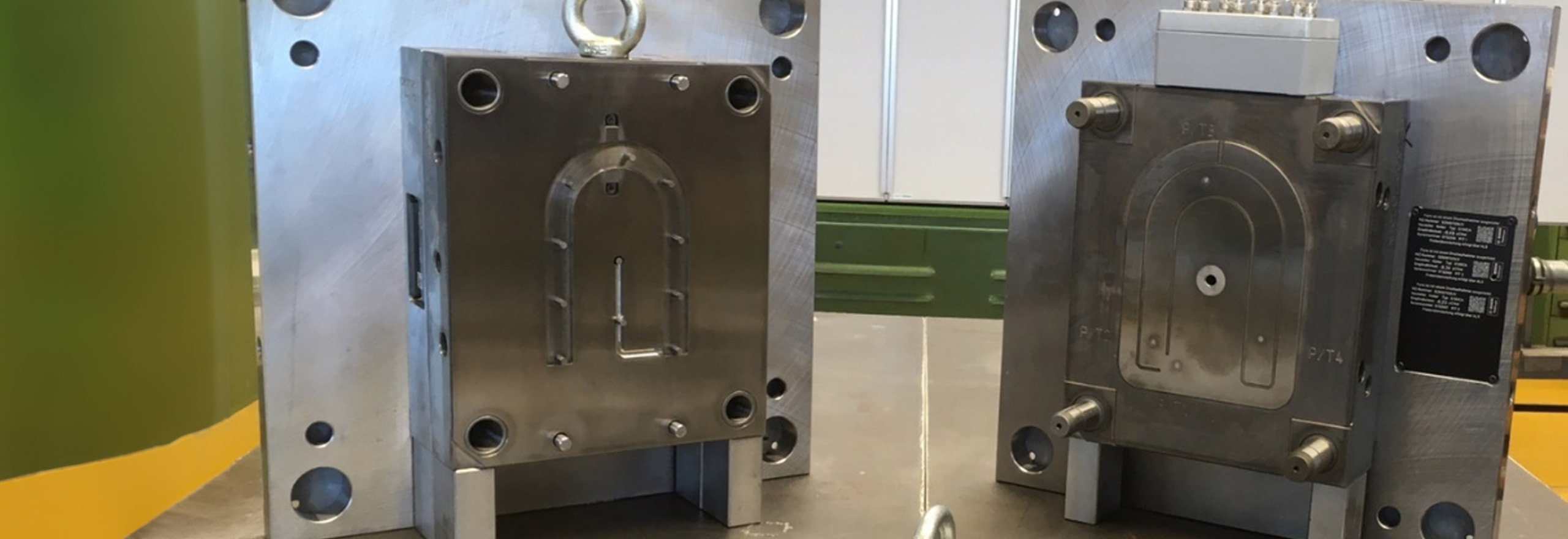
Engineering Reality 2024 volume 1
Accelerate Smart Manufacturing
The Bosch Group is a leading international technology and service company with €88.2 billion in sales revenue and 421,300 associates worldwide. With innovative and inspiring products and services, Bosch improves the quality of life of people around the world. Through its 468 subsidiaries and regional companies, Bosch has a presence in more than 60 countries.
The company’s activities divide into four divisions: Mobility Solutions, Industrial Technology, Consumer Goods, and Energy and Building Technology. Its strategic goal is to create solutions and products for connected life that either have artificial intelligence (AI) or are developed or manufactured with its help.
Operating in a global network, Bosch Research fills the innovation pipeline of the Bosch business sectors. The company uses plastic components across every business sector, including gear wheels for e-bikes or robotic lawnmowers. One recent Bosch Research project focused on industrializing engineering methods for the virtual design of injection moulded plastics. Bosch tasked its central research division to create credible simulation workflows that all engineering teams across the world can easily use.
Crystallisation of semi-crystalline thermoplastics makes simulating shrinkage and warpage challenging
In the traditional design process at the different engineering departments, simulation is used to predict the impact of design parameters like gate location, and process parameters like mould temperature on the injection pressure and aftermoulding warpage.
In terms of polymer materials, Bosch primarily uses high-performance engineering thermoplastics and most of them are semi-crystalline ones. During the injection moulding of semi-crystalline thermoplastics, flow-induced crystallisation takes place and influences the viscosity and solidification of the material during processing. This behaviour is crucial as it impacts the pressure need during the injection moulding process, and the final part’s shrinkage and warpage.
The type of crystallisation that happens during the injection moulding process is complex and challenging to model mathematically. Additionally, the available models in injection moulding software are difficult to calibrate experimentally, with many variables that are expensive to identify for each single material. For design purposes, however, engineers need a way to simulate semi-crystalline materials without oversimplifying crystallisation.
Since neglecting or simplifying the crystallisation phenomenon impacts the simulation results, our team at Bosch Research was keen to close the gap and achieve a more robust simulation framework for semi-crystalline thermoplastics.
Implementing a flow-induced crystallisation model into injection moulding simulation
One course of action to improve the accuracy of the virtual engineering tasks for semi-crystalline thermoplastic parts is to implement a crystallisation model into the injection moulding simulation software tools. One challenge here is that such a model should be able to describe the crystallisation process under the thermo-mechanical conditions of the injection moulding process, which exhibits high cooling rates (up to hundreds of K/s) and high shear rates (up to thousands 1/s). In particular, the deformation conditions of the melt have non-negligible effects on the crystallisation kinetics of a semi-crystalline polymer. This phenomenon is known as flowinduced crystallisation.
At this point, we had to choose between two kinds of modelling approaches: A phenomenological model describing the nucleation and growth of the crystalline phase, or a thermo-mechanical model developed in the framework of generalized standard materials. The first modelling approach is well established within the research community but requires a costly and time-consuming parameter identification. In addition, including the effect of flowinduced crystallisation increases the number of material parameters, and the material parameter identification is performed at conditions far away from the actual injection moulding ones. The second modelling approach, which is founded on irreversible thermodynamics, is more exotic within the polymer processing research community but exhibits some advantages over the phenomenological approach: It intrinsically includes the effect of flow-induced crystallisation and has a considerably lower number of material-dependent parameters.
Given the previous reasons, we decided to implement the thermo-mechanical based model as user-defined routine into a commercial software for injection moulding simulation. The implementation also included a new term in the energy equation accounting for the latent heat of crystallisation as well as crystallisation-dependent models for viscosity, density (or PVT) and solidification. At this stage, the open task was to establish a reliable method for the identification of the material-dependent parameters. ODYSSEE entered here to perform this task in a smart fashion.
Accurate surrogate models for parameter identification
As mentioned before, the flow-induced crystallisation in injection moulding occurs at thermo-mechanical conditions that are extremely difficult to recreate even with the cutting-edge material characterization equipment. To overcome this obstacle, our R&D team planned to perform the parameter identification using data acquired directly from injection moulding trials. Concretely, we proposed to use in-mould pressure signals as indirect descriptor of the crystallisation phenomenon and reference data for the calibration of the implemented models.
A parameter calibration is fundamentally an optimisation problem performed over a mathematical function. The task is to find out the values of the input variables that minimize the error between the function output and a reference of that output, which is typically obtained experimentally or by alternative model approaches. In our case, the function is the high-fidelity simulation model with our user-defined implementations, the input variables are the unknown material parameters of the flow-induced crystallisation model, the outputs are the in-mould pressure signals computed at the sensor locations, and the reference is the actual pressure signal acquired by the mould sensors.
Optimisation algorithms normally require the repeated evaluation of the function in a loop to reach an acceptable local minimum or maximum. On the other hand, the number of required iterations increases substantially with the number of independent variables to optimise. The problem here is that our high-fidelity injection moulding simulation is a computationally expensive function, which requires minutes (even hours) to generate an output, and so the optimisation could easily become an extremely time-consuming process. To solve this issue, ODYSSEE CAE comes into play. Basically, with the help of ODYSSEE we can generate surrogate models (or metamodels), which are able to emulate the results of the high-fidelity simulation, but with a considerably lower computational cost. Instead of the high-fidelity simulation, we can use those surrogate models within the optimisation routine and identify the material parameters of the flowinduced crystallisation model in a matter of seconds.
The value added of ODYSSEE is the smooth and robust workflow for generating surrogate models based on input/ output (I/O) data. For our case, ODYSSEE even provides tools for creating a suitable Design of Experiment (DoE), which is the basis for sampling output data by evaluating the high-fidelity simulation in the space of the input variables. The generation of surrogate models is in principle a supervised machine learning problem that ODYSSEE has implemented using the classical technique of splitting I/O data into training and validation datasets for evaluating the performance of the surrogate model. Once the input/output data are loaded in ODYSSEE, the tool allows to compare all surrogate modelling approaches, which includes reduced order models (ROM) and advanced interpolation methods, making the selection of the most pertinent surrogate model technique more straightforward.
Finally, by using the actual pressure signals acquired by the mould sensors as target, we can also execute optimisation tasks inside ODYSSEE using the previously created surrogate models. Interestingly, with the help of the Hexagon team we could customize the optimisation algorithm for this particular use case of material data identification. With the materialdependent parameters identified at the surrogate model level, we can now use them at the high-fidelity simulation level for computing any other geometry using the same material. The figure above shows the improved accuracy in pressure estimation of the high-fidelity simulation with crystallisation model (calibrated using ODYSSEE) versus the default highfidelity simulation (without crystallisation model). The root mean square error should be zero for the highest accuracy, while the coefficient of determination should tend to 1 when the accuracy increases.
Figure 1: Performance of the Default vs. calibrated crystallization (Optimization) model in the pressure estimation of the high-fidelity injection molding simulation
Metamodel-based parameter identification reduces development time and costs
Using ODYSSEE metamodel optimisation, we found a smart method for identifying material-dependent parameters from data acquired under actual injection moulding conditions. This efficient parameter calibration is a significant contribution for deploying our flow-induced crystallisation model within an industrial high-fidelity injection moulding simulation software. In doing so, we take a huge step towards a more accurate estimation of pressure needs and warpage in injection moulding simulation.
This new approach of parameter identification has helped the company to save money and time. Replacing the traditional route of material characterization and identification by the novel approach of actual-process data-driven calibration using a metamodel reduces time effort by 90% and slashes costs by 60%. With the consideration of flow-induced crystallisation model into the injection moulding simulation, Bosch expects substantially reduce the problems at the shop floor related with short shots or incomplete mould filling.
With the help of this enhanced process simulation, the engineering departments will be able to adjust their design geometries with higher confidence and/or define more accurately the machine pressure requirements to avoid costly and time-consuming mould recursions or trial and error procedures at the shop floor. In short, Bosch aims to reduce the development time of injection-moulded parts by enabling a more robust and accurate virtual design phase.
A user-friendly interface eases industrialization
To guarantee an industrial deployment of the metamodel-based parameter identification, we require software tools with a high level of UX maturity. ODYSSEE is a good choice in our workflow because it has a user-friendly interface for loading data, training and evaluating different surrogate modelling approaches, and finally performing optimisation runs.
After validating the concept of the metamodel-based parameter identification with a polyoxymethylene material, we put an industrial workflow in place for assuring the calibration of any other semi-crystalline thermoplastic material. This ensures an industrial pipeline for efficiently providing material data to the whole spectra of plastics engineering departments of the Bosch group around the world.
The industrial deployment of the high-fidelity simulation with flow-induced crystallisation model is depicted in Figure 2. The first 3 steps correspond to the material parameters identification assisted by ODYSSEE. Those steps occur within Bosch Research (Central R&D division) for all new materials. In this offline stage, we obtain basically material cards for our flow-induced crystallisation model, which are then made available to all simulation engineers of the Bosch group.
Step 4 represents essentially the day-to-day injection moulding simulation including flow-induced crystallisation, which needs the material cards identified with the help of ODYSSEE. For the simulation engineers within the different divisions of Bosch, the additional effort is limited to activating the user-defined solver API and load the respective material card. Just a few more clicks to benefit from a more reliable estimation of pressure development and final warpage in injection moulding.
Figure 2: From concept to industrialization – Injection molding simulation with flow-induced crystallization model at Bosch
This successful use case of metamodel-based optimisation is a steppingstone for extending the application of those techniques to other research processes. Bosch set a mandate indicating that the company looks for new domains of application where the use of surrogate models, machine learning, and artificial intelligence deliver an engineering advantage.