HxGN Visual Detection: Machine Learning
AI-powered surface inspection
Product enquiry
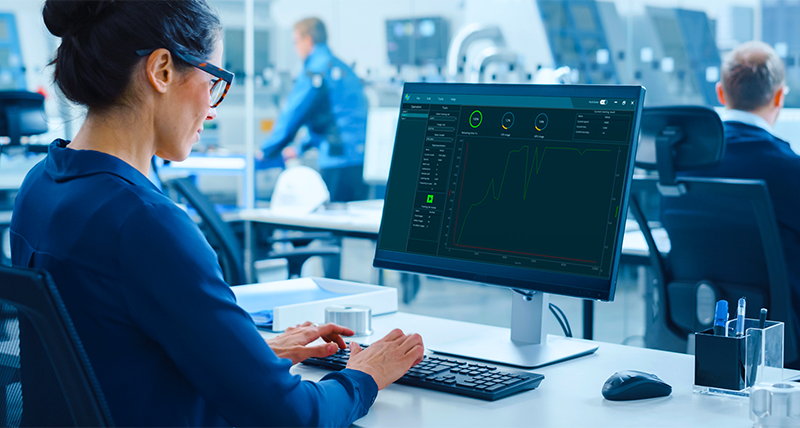
HxGN Visual Detection uses CNN (convolutional neural network) deep learning models to train, identify and categorise surface defects. It requires a relatively small quantity of defects samples (in the order of hundreds) to start. As it uncovers undetected defects, the model can be trained to further its ability to identify true positives.
The algorithms in HxGN Visual Detection employ pattern recognition, statistics, deep learning and other image processing techniques to rapidly learn about surface deviations.
The HxGN Visual Detection machine learning process
1. Label
2. Train
3. Inspect
Deep learning drives greater accuracy
Successful deep learning relies on the depth and sensitivity of the learning model and its environment. HxGN Visual Detection includes a set of models (X-CNN, X-CNN-Tiny, X-CNN-Plus and Segmentation) which are optimised for performance and accuracy.
The construction of an advanced neural network increases HxGN Visual Detection’s ability to quickly and correctly identify defects, also known as true positives. Powerful algorithms including synthetic image data augmentation, image morphing, normalisation and dimensionality reduction all contribute to this process.
The foundations of these models are based on accepted industry frameworks such as GAN, YOLO, and RCNN.
HxGN Visual Detection provides an open architecture design that can be easily integrated with custom CNN models. The application is extendable through an API and can be integrated into third party automated manufacturing cells.
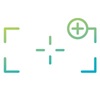
Advanced neural networks
Synthetic image data augmentation, image morphing, and normalisation algorithms.
Easy to use hyperparameters
Fine-tuned image analysis without coding.
Built on YOLO (you only look once) CNN principles
Leading neural network for fast object detection.
Choose from a range of CNN models
Select the most useful machine learning model for your application.
Automate with third-party systems
Add to vision and metrology workflows via Bridge application.