HxGN Visual Detection: Intelligenza Artificiale
Ispezione della superficie basata sull'IA
Richiesta di informazioni sui prodotti
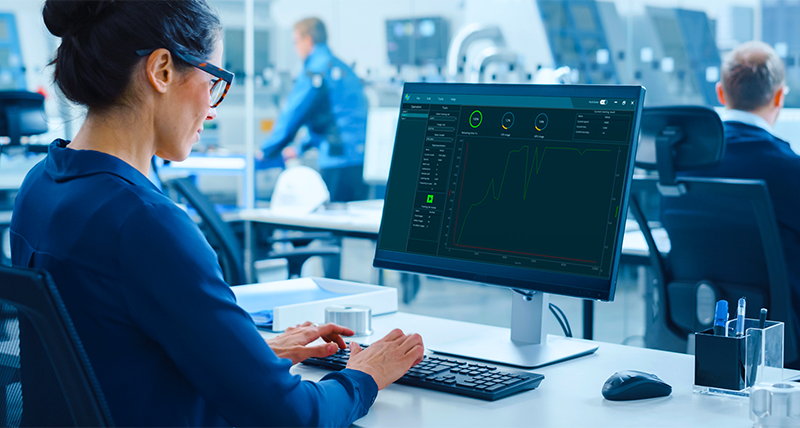
HxGN Visual Detection utilizza modelli di apprendimento profondo CNN (rete neurale convoluzionale) per addestrare, identificare e classificare i difetti superficiali. Richiede una quantità relativamente piccola di campioni di difetti (in ordine di centinaia) per iniziare. Poiché individua i difetti non rilevati, il modello può essere addestrato per migliorare la sua capacità di individuare i veri positivi.
Gli algoritmi di HxGN Visual Detection utilizzano il riconoscimento dei modelli, la statistica, il deep learning e altre tecniche di elaborazione delle immagini per apprendere rapidamente le deviazioni della superficie.
Il processo di apprendimento automatico di HxGN Visual Detection
1. Etichetta
2. Settore ferroviario
3. Inspect
Deep learning drives greater accuracy
Successful deep learning relies on the depth and sensitivity of the learning model and its environment. HxGN Visual Detection includes a set of models (X-CNN, X-CNN-Tiny, X-CNN-Plus and Segmentation) which are optimised for performance and accuracy.
The construction of an advanced neural network increases HxGN Visual Detection’s ability to quickly and correctly identify defects, also known as true positives. Powerful algorithms including synthetic image data augmentation, image morphing, normalisation and dimensionality reduction all contribute to this process.
The foundations of these models are based on accepted industry frameworks such as GAN, YOLO, and RCNN.
HxGN Visual Detection provides an open architecture design that can be easily integrated with custom CNN models. The application is extendable through an API and can be integrated into third party automated manufacturing cells.
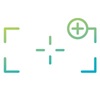
Advanced neural networks
Synthetic image data augmentation, image morphing, and normalisation algorithms.
Easy to use hyperparameters
Fine-tuned image analysis without coding.
Built on YOLO (you only look once) CNN principles
Leading neural network for fast object detection.
Choose from a range of CNN models
Select the most useful machine learning model for your application.
Automate with third-party systems
Add to vision and metrology workflows via Bridge application.